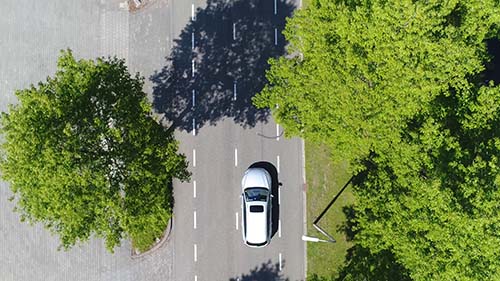
By Yao-Ching Yu (AI Research Engineer)
ChatGPT is fueling the imagination of the public with its endless applications. Even the automotive industry is picking up on this excitement as, historically, autonomous vehicles have directly benefited from the latest AI breakthroughs.
In the past few years, OpenAI has been introducing AI models. The most notable of these are Generative Pre-trained Transformer (GPT-3), a language model; ChatGPT’s predecessor released in 2021, DALL-E, a deep learning model that generates images based on written prompts; and most recently, ChatGPT, a chatbot based on an updated language model and released in 2022.
ChatGPT’s conversational AI allows users to interact with the model as it fulfills their requests. ChatGPT’s dialogue format enables it to answer follow-up questions, reject inappropriate requests, admit mistakes, and challenge incorrect statements, among other dynamic responses.
Functionally, ChatGPT can complete tasks such as writing emails and video scripts, translating, coding, and copyediting, using intelligence far beyond all current human-machine interaction models. The clamor that ChatGPT has inspired has placed unprecedented pressure on search giants such as Google and Baidu, which have tried to keep up by releasing ChatGPT-like models, although the effectiveness of these models has yet to be reported.
What can ChatGPT, having reached such an advanced level of intelligence, bring to the autonomous driving industry? Does it signal breakthroughs in the decision-making problems that have plagued practitioners for years? What does this mean for automotive security?
The role of AI in the history of autonomous vehicles
To answer the above questions, it is best to understand why advancements in AI technology also draw attention to developments in autonomous driving. By simply reviewing the history of autonomous driving, one can see that every breakthrough in the industry is synchronized with the development of AI technology.
As commonly understood, AI imitates the neural networks of the human brain. It learns very humanlike skills by analyzing large amounts of data. As far back as the 1980s, the first practical application of neural networks occurred in autonomous driving.
In 1987, researchers at the Carnegie Mellon University Artificial Intelligence Laboratory attempted to develop a self-driving truck. To do so, they manually programmed all driving behaviors and wrote detailed instructions for all situations encountered on the road to make the vehicle drive automatically. While this approach allowed the vehicle to move, it did so only at the rate of a few inches per second.
Since manual coding did not work, a doctoral student, Dean Pomerleau, chose another approach: the neural network method. He created the Autonomous Land Vehicle in a Neural Network system, or ALVINN for short. In creating ALVINN, he used a truck with a rooftop camera to track what drivers were doing. ALVINN learned to drive by observing how drivers navigated roads. By the early 1990s, ALVINN was able to reach speeds of up to 70 miles per hour.
Breakthroughs in computer vision models
Aside from ALVINN, breakthroughs in computer vision models were key to autonomous driving. In 2012, Professor Jeff Hinton and two of his students, Alex Krizhevsky and Ilya Sutskever, won the ImageNet image recognition competition and published a paper introducing the algorithm AlexNet. This paper was a turning point not only for AI but also for the global technology industry.
Object detection and image recognition are also key technologies for autonomous driving, and the industry has benefited from breakthroughs in computer vision algorithms. Therefore, when Dr. Kai Ming He’s algorithm achieved accuracy in image recognition that for the first time surpassed human performance in 2015 on Stanford AI Lab Director Fei-Fei Li’s ImageNet open dataset, autonomous driving also entered the fast lane of development.
Is ChatGPT an AI milestone for autonomous driving?
Given that autonomous driving is one of the direct applications of AI implementation, we now go back to the question of ChatGPT. Two things would need to be clarified:
- Does autonomous driving refer to low-level autonomous driving (assisted driving) or high-level or Level 4 autonomous driving (fully autonomous)?
- Is ChatGPT a language model or a more generalized generative model?
By answering these questions, we can see that as a natural language model, ChatGPT may have a more direct impact on human-machine interaction in assisted driving than on Level 4 autonomous driving.
ChatGPT and human-machine interaction
In human-machine interaction, ChatGPT still opens exciting direct applications for vehicles. In fact, there is no interaction more efficient than voice interaction, whether compared to gesture interaction or button interaction. For example, by using ChatGPT, vehicles can interact with drivers through voice or text and provide real-time feedback on vehicle status, driving information, and more.
Before this, although there were already many in-car interaction systems, the industry’s pain points focused on understanding responses. Most in-car voice interaction systems were not intelligent enough to fully understand user responses, resulting in limited system functions and few command words. ChatGPT’s performance has given the market hope for a solution.
Imagine this scenario: drivers chatting with their cars to pass the time on the road. Not a foreign concept at all, since this scenario has certainly been depicted in popular media for years. In the future, ChatGPT can tell jokes to drivers and use a more natural communication style that is more human than machine.
ChatGPT is a sign of more AI milestones to come
Looking at generative models more broadly, models with large amounts of data and large parameters can help achieve higher levels of autonomous driving. Vehicle capabilities mainly include perception and cognition, with perception relying heavily on computer vision and cognition relying more on generative techniques like ChatGPT. Therefore, the revolutionary significance of ChatGPT is that it has ushered in an era of knowledge and reasoning for AI models. Currently, the biggest shortcoming of autonomous driving is the lack of sufficient intelligence in decision-making and planning.
ChatGPT uses a training method called reinforcement learning from human feedback (RLHF). This method first trains a reward model based on human feedback to determine whether the model’s output is satisfactory to humans. During training, this pretrained reward model is used to score the output of the model being trained, such as GPT-3, instead of relying on real human judgment.
The introduction of this method allows for heavy use of the reward model to simulate human feedback during training, ensuring the minimization of useless, distorted, or biased information in the output. In autonomous driving decision-making algorithms, there is a type of learning called imitation learning, which teaches machines how human drivers behave in different scenarios.
The success of ChatGPT is undoubtedly exciting for professionals in the autonomous driving industry, as it demonstrates the extent to which machines can learn human knowledge and validates the effectiveness of RLHF.
Every takeover by a human driver is human feedback on the autonomous driving strategy. This takeover data can be used simply as a negative sample, which records when the autonomous driving decision-making is corrected. It can also be used as a positive sample to improve cognitive decision-making.
This means that if the idea of human feedback reinforcement learning is also adopted in the development of autonomous driving, a reward model can be trained to verify and evaluate the output of autonomous driving models, allowing them to continuously improve and eventually reach the level of human driving.
Coupled with the excellent generalization ability inherent in large models, it may eliminate corner cases, rare events that are possible when a person is driving but have a very low frequency of occurrence in autonomous driving. Although it is rare in daily life, encountering a corner case that cannot be resolved by the autonomous driving system might lead to fatal traffic accidents. In fact, before ChatGPT, Tesla in the US and Baidu in China were already exploring the route of large-parameter models.
In the second installment of our two-part discussion of ChatGPT, we tackle its impact on automotive security and safety.
Learn more about automotive security by visiting our homepage.